Reliable microelectronics through failure analysis with artificial intelligence
The use of machine learning methods offers novel possibilities for automation, and thus for increasing the efficiency of failure diagnostic methods for electronic components and systems. Together with partners, the Fraunhofer Institute for Microstructure of Materials and Systems IMWS in Halle (Saale) wants to pioneer the way. The new methods are intended to facilitate the detection and analysis of complex failure modes. Furthermore, increasingly automated failure analysis and defect categorization will allow more components and data to be analyzed in order to detect and eliminate quality problems that have been undetectable up to now. This allows the production quality and lifespan of the components to be increased even further.
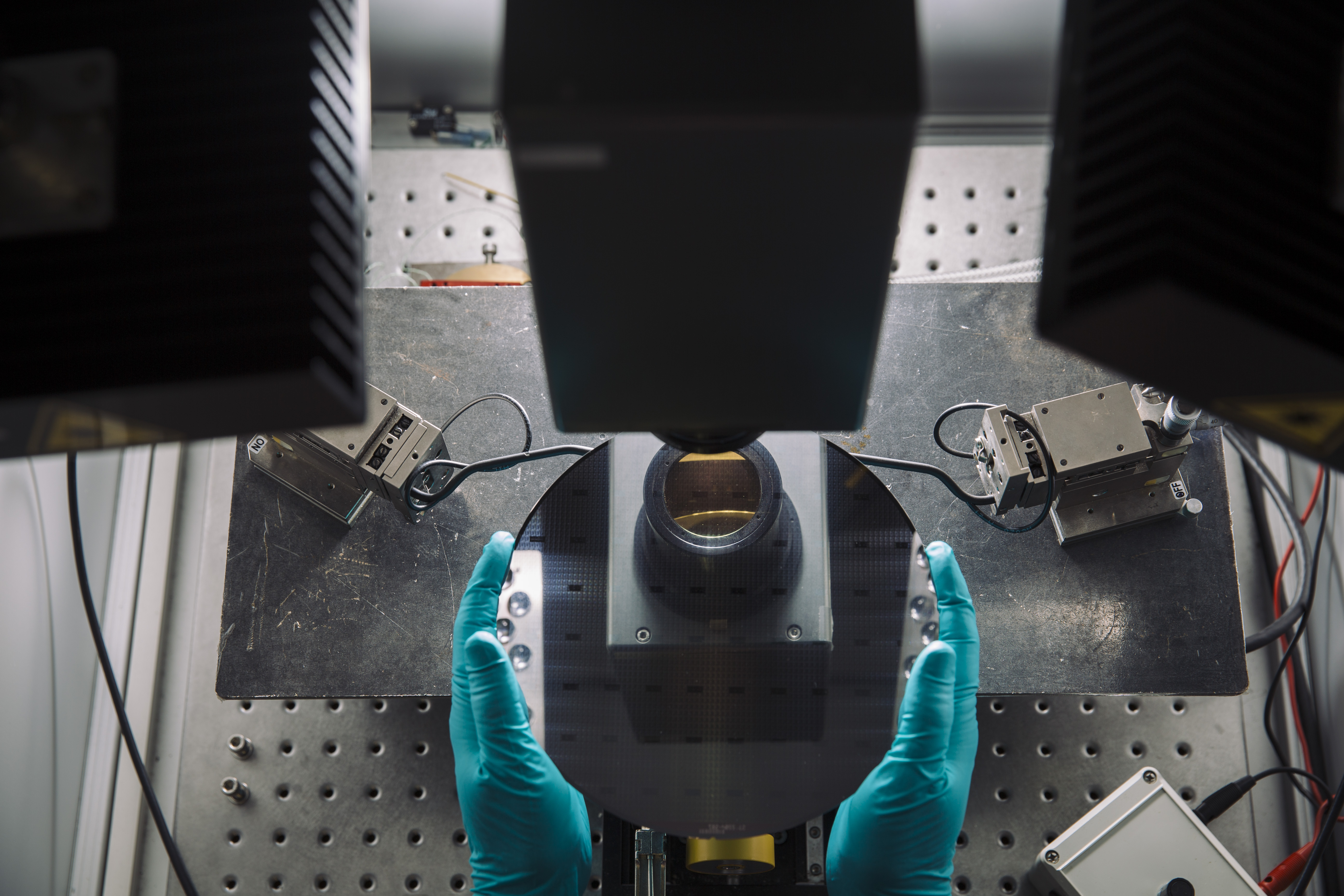
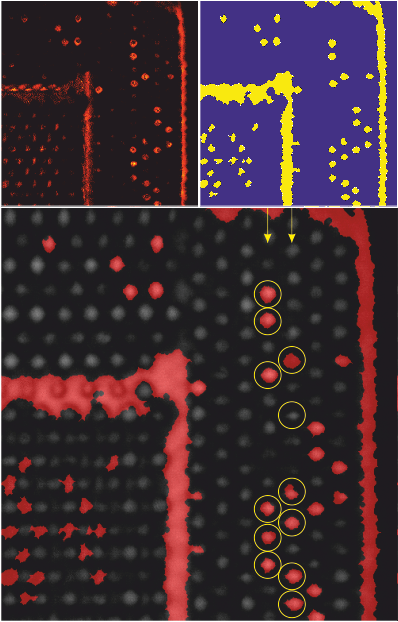
Whether it's about new mobility, intelligent production, or modern energy technology: reliable and robust electronics are a prerequisite in all of these areas for long-term success in the market. “There a general trend in electronic components towards integrating more and more functionality into smaller and smaller packages. This high integration density is a constant challenge to the quality and reliability of the components. If you want to find out – regardless of whether it’s during production, product qualification, or on a product returned by the customer – where which defect is located and what caused it, then more sophisticated technologies are always necessary”, says Frank Altmann, who heads the activities of the Fraunhofer IMWS in the new project. “Powerful and constantly improved methods for failure analysis are therefore of fundamental importance. On the one hand, this will help the industry develop reliable electronic products. On the other hand, in applications such as autonomous driving, communication technology, or medical applications, it must be ensured that the electronics will continue to operate reliably under the particular environmental conditions.”
The Fraunhofer IMWS is already helping the electronics industry find answers to their questions, in particular through its deep understanding of degradation mechanisms and the causes of material failures, and develops a variety of methods for failure analysis and material characterization. In cooperation with our partners in the project “Failure Analysis 4.0 – Key for reliable electronic devices in smart mobility and industry production”, our goal is to make even more efficient failure analysis tools and workflows available. In this project, the project partners want to use methods of artificial intelligence (AI) to enable faster and better detection and classification of defects.
The project with the component manufacturers BOSCH, Infineon, ST-Microelectronics, and ERICSSON, the diagnostic device manufacturers PVA TePla AS, CyberTECHNOLOGIES, KERN Mikrotechnik, Matworks, TESCAN/Orsay, Gimic AB, Excillum, and Direct Conversion as well as research facilities at the University of Stuttgart, Ecole de Mines, University of Saint-Etienne, and the Research Institutes of Sweden (RISE) runs until March 2023. “We want to utilize the enormous potential of AI-based methods for almost fully automated failure analysis and data handling based on the concept of Industry 4.0. This will significantly improve the performance of analyses, the precision, and the success rate when troubleshooting and determining the causes of failures”, says Altmann.
The Fraunhofer IMWS focuses on the research and development of AI methods to analyze the signals generated by non-destructive defect detection methods like scanning acoustic microscopy (SAM) and lock-in thermography (LIT). The goal is to develop concepts for monitored as well as unmonitored machine learning and integrate them into diagnostic devices for data acquisition and evaluation.
Various advantages would be gained if it were possible to link data obtained using different analysis methods such as electrical measurements, material characteristics, defect localization methods, microstructural analysis methods, and the layout of an integrated circuit and then analyze the data using a correspondingly trained and self-learning algorithm. This would allow defects to be detected automatically and their signatures to be determined, classified, and compared with existing failure catalogs – this would then substantially reduce the time required for failure analyses, standardize the analysis workflow, and reduce the subjective influence of humans on the results of the analyses. “This is especially important for complex failure modes that occur very rarely but can still lead to serious impairment of the function of electronic components”, says Altmann.
In order to realize this holistic approach to failure analysis workflows, though, numerous research questions need to be answered in the project. For example, individual analysis devices from various stages of the process need to be connected to each other using standardized hardware and software interfaces, guidelines and database systems for structuring, processing, and storing component-specific characteristic and analysis data need to be defined, new AI-enhanced analysis methods need to be created, and AI-based algorithms need to be developed.
Altmann summarizes as follows: “In the current failure analysis workflows, numerous methods that provide additional, complementary component analysis data are used, and this data usually needed to be evaluated manually up to now. The automated, cross-process provision and AI-based evaluation of this data, including determination of the causes of failure, has enormous potential for further increasing the efficiency of quality controls used in production, and thus for increasing product reliability and quality in spite of increasingly shorter development cycles.”