Quality assurance is a key enabler for modern microelectronics which is only possible if powerful techniques for screening, fault isolation and failure diagnostics are available. Machine learning (ML) and artificial intelligence (AI) can strongly support these tasks, however versatile applicable data infrastructures and evaluation algorithms are still not readily available. In the "FA2IR" project, the Fraunhofer Institute for Microstructure of Materials and Systems IMWS in Halle (Saale) is working with partners on solutions to develop and incorporate sophisticated digital tools for paving the digital path for fault analysis. Automated, AI-supported analysis is intended to minimize result-subjectivity, shorten development times and to improve product quality.
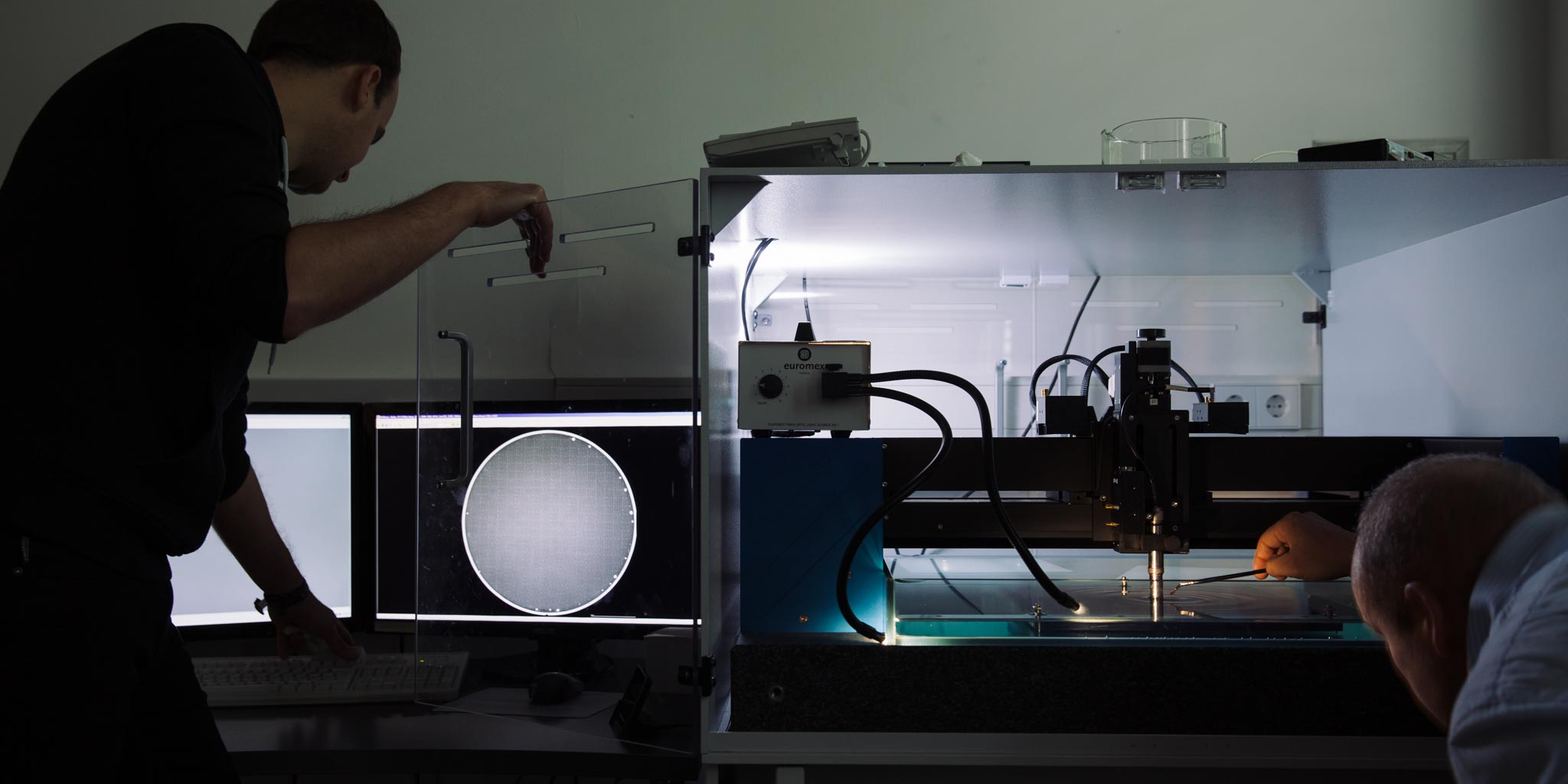
Autonomous driving, sustainable energy systems, modern medical equipment: it all requires high-performance microelectronics. Innovative technological concepts, the associated manufacturing processes and materials and material combinations are opening up new horizons. However, these changed conditions also harbor the risk of novel defect types and failure mechanisms, from which entirely new challenges arise with respect to the materials science impacting the assurance of reliability and quality assessment. A key focus here is on the investigation of material interactions aiming at the optimization of both the technologies and their manufacturing processes. As microelectronics continues to develop, the techniques of failure analysis and quality assessment are required to keep pace.
"Modern electronic systems are becoming ever more complex, smaller and more powerful and effective analysis techniques are essential for them to succeed in the market. They are necessary for the manufacturers as early as in the product development stages to investigate material behavior and subsequent defect affinity to ensure that the next-generation products exhibit the desired performance, robustness and reliability over their entire life span," says Dr. Sebastian Brand, Team Leader "Non-destructive Diagnostics" at the Fraunhofer IMWS.
ML and AI methods will be part of the solution for overcoming these challenges efficiently. Instead of the more manual failure analysis and interpretation of measurement data based on individual experience that is common today, the use of AI-based algorithms allows data to be automatically interpreted, evaluated and inter-linked - resulting in a largely increased efficiency and precision.
AI and ML have evolved to provide powerful approaches in recent years, so they can considerably add value, especially in data analysis tasks. However, their potential cannot yet be fully exploited due to the absence of a standardized infrastructure for data management and the necessary handling as well as the corresponding training data. "In previous projects, we were able to demonstrate the value of deep learning approaches for the non-destructive characterization of materials and the gaining of a deep understanding of degradation mechanisms and the subsequent defect formation. Unfortunately, there is still a lack of further data for enabling a widespread application. In addition, specific evaluation algorithms, standardized interfaces for communication and data exchange between different diagnostic tools or suitable cloud computing systems for a secure and fast evaluation and use of large amounts of complex data are not available to the extent required," says Brand.
This is where the European XCES project "Failure Analysis - AI-Readiness and Application (FA2IR)" aims to create the appropriate solutions. The project, which runs until the end of 2026 and which is funded by the German Federal Ministry of Education and Research, aims to pave the ground for the holistic use of machine learning for failure analysis. One goal is the consistent and coordinated digitalization of all analysis processes and process steps, solutions for data management and intra-tool communication with a generally accepted format for meta and raw data and standardized interfaces as well as the establishment of comprehensive databases with reliability-relevant material and failure information. Another central component is the automated interpretation of measurement data and analysis results supported by AI methods and their linkage with existing knowledge, that is made available in the form of failure analysis databases.
The project partners aim to create a comprehensive and fully integrated environment for managing and processing digital data, algorithms, methods and models for machine learning that can be applied globally and dynamically expanded to new data and cases. To this end, Infineon, Bosch, Zeiss, PVA TePla AS GmbH, Matworks GmbH, the University of Stuttgart and Fraunhofer IWMS have pooled their expertise, along with partners from Sweden, the Netherlands and France.
Brand describes a scenario for future solutions: "Data is derived from existing failure catalogs, databases and literature in a defined format using natural language processing and made available to the failure analysis engineer as a knowledge base. This allows known failure modes and degradation mechanisms to be identified and verified much faster. Measurement data such as electrical characteristics, signal traces, spectra and microscopy images can be combined and defects can be identified faster and with an increased reliability in an semi-automated manner. The ultimate goal is the development and training of machine learning models that have the ability to identify defects and their causes in microelectronic samples with minimal analysis effort and personnel input. Another major advantage of such approaches is the reduction of the influence of human subjectivity and experience on data interpretation. Obtained expertise and knowledge can be applied more easily, even by untrained personnel."
The automation of defect detection, localization and classification can make a significant contribution towards the reduction of subjectivity in the interpretation of complex and multidimensional measurement data. This is one of the focal points of the Fraunhofer IMWS project. Therefore, particular attention is paid to the signal analysis and evaluation of non-destructively operating methods such as acoustic microscopy and lock-in thermography.
"I am convinced that AI will bring better and faster results in failure diagnostics. The solutions we are striving for can enable shorter development times, faster market launches and improved product quality, especially for complex failure modes. This is an important prerequisite for ensuring that European industry remains competitive in important technological fields of the future and that novel technologies will be accepted due to their proven reliability." says Brand.
(January 23, 2025)